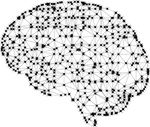
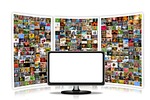
import pandas as pd
import numpy as np
import talib
from sklearn.tree import DecisionTreeClassifier
from sklearn.model_selection import train_test_split
def extract_features(df):
# Calculate RSI
df['rsi'] = talib.RSI(df['close'].values, timeperiod=14)
# Calculate Bollinger Bands
upperband, middleband, lowerband = talib.BBANDS(df['close'].values, timeperiod=14)
df['bollinger_upper'] = upperband
df['bollinger_middle'] = middleband
df['bollinger_lower'] = lowerband
# Calculate Moving Average
df['ma50'] = talib.SMA(df['close'].values, timeperiod=50)
return df
def label_data(df):
# Mark the rows where the RSI value is above 70 as "divergence" (0)
# Mark the rows where the RSI value is below 30 as "convergence" (1)
df['label'] = np.where(df['rsi'] > 70, 0, 1)
df['label'] = np.where(df['rsi'] < 30, 1, df['label'])
return df
def load_data(filename):
df = pd.read_csv(filename)
df = extract_features(df)
df = label_data(df)
return df
def train_model(df):
# Split data into training and testing sets
X = df.drop(['label'], axis=1)
y = df['label']
X_train, X_test, y_train, y_test = train_test_split(X, y, test_size=0.33, random_state=42)
# Train a decision tree model
clf = DecisionTreeClassifier(random_state=0)
clf.fit(X_train, y_train)
# Test the model on the testing data
accuracy = clf.score(X_test, y_test)
print("Accuracy:", accuracy)
return clf
def predict(clf, df):
# Use the trained model to predict the labels for the test data
X = df.drop(['label'], axis=1)
y_pred = clf.predict(X)
return y_pred
# Load the data from a CSV file
df = load_data('stock_data.csv')
# Train the model
clf = train_model(df)
# Use the trained model to predict the labels for new data
y_pred = predict(clf, df)
import pandas as pd
import numpy as np
from sklearn.tree import DecisionTreeClassifier
from sklearn.metrics import accuracy_score
import joblib
def load_data(file_name):
df = pd.read_csv(file_name)
return df
def preprocess_data(df):
df = df.dropna()
df['rsi'] = calculate_rsi(df)
df['bolinger_band'] = calculate_bolinger_band(df)
df['ma50'] = calculate_ma50(df)
return df
def calculate_rsi(df):
gain = df['close'].diff().where(df['close'].diff() > 0, 0)
loss = df['close'].diff().where(df['close'].diff() < 0, 0)
avg_gain = gain.rolling(14).mean()
avg_loss = loss.rolling(14).mean().abs()
rs = avg_gain / avg_loss
rsi = 100 - (100 / (1 + rs))
return rsi
def calculate_bolinger_band(df):
rolling_mean = df['close'].rolling(window=20).mean()
rolling_std = df['close'].rolling(window=20).std()
bolinger_high = rolling_mean + (rolling_std * 2)
bolinger_low = rolling_mean - (rolling_std * 2)
bolinger_band = (df['close'] - bolinger_low) / (bolinger_high - bolinger_low)
return bolinger_band
def calculate_ma50(df):
ma50 = df['close'].rolling(window=50).mean()
return ma50
def create_features_and_labels(df):
df['price_pattern'] = np.where(df['close'].diff(1) > 0, 1, 0)
df['rsi_pattern'] = np.where(df['rsi'].diff(1) > 0, 1, 0)
X = df[['rsi_pattern', 'bolinger_band', 'ma50']]
y = df['price_pattern']
return X, y
def train_model(X_train, y_train):
model = DecisionTreeClassifier()
model.fit(X_train, y_train)
return model
def evaluate_model(model, X_test, y_test):
y_pred = model.predict(X_test)
accuracy = accuracy_score(y_test, y_pred)
return accuracy
def save_model(model, file_name):
joblib.dump(model, file_name)
def load_model(file_name):
return joblib.load(file_name)
def write_result_to_csv(file_name, stock_name, result):
with open(file_name, 'a') as f:
f.write(f'{stock_name},{result}\n')
if __name__ == '__main__':
stock_
# Get a list of all the CSV files in the directory
files = [f for f in os.listdir() if f.endswith('.csv')]
# Read each file into a separate DataFrame and store them in a list
dfs = [pd.read_csv(f) for f in files]
# Concatenate the DataFrames into a single DataFrame
df = pd.concat(dfs, ignore_index=True)
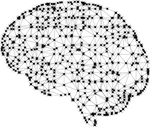
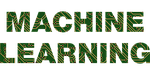
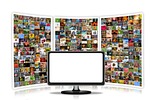